Advancing Network Security: Carleton's Best Poster Runner-Up at IEEE CNS
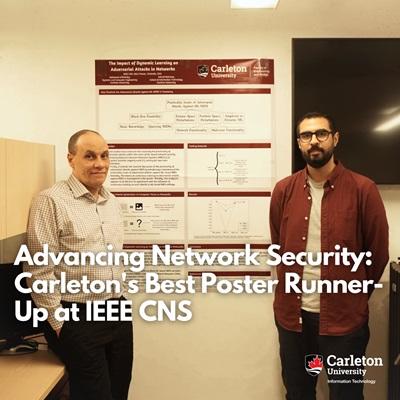
December 12th, 2023
We are thrilled to announce that the groundbreaking research conducted by SCE's outstanding Ph.D. student, Mohamed ElShehaby, under the guidance of Dr. Ashraf Matrawy, has been recognized at the IEEE Conference on Communications and Network Security (CNS) 2023 and secured the prestigious Best Poster Runner-Up accolade at this esteemed event.
Mohamed ElShehaby has been spearheading our investigations into adversarial machine learning (ML) attacks within network environments. In response to the growing ubiquity of ML in Network Intrusion Detection Systems (NIDS), Mohamed was tasked with assessing the practicality of adversarial attacks in network security, drawing comparisons to their more well-studied counterparts in computer vision.
Ongoing Work: Explore the ongoing research on adversarial attacks in ML-based NIDS by Mohamed ElShehaby. View the comprehensive work posted on arXiv.
Machine Learning has revolutionized the field of cybersecurity, particularly in the realm of NIDS, offering automated and highly accurate processing of vast amounts of data. However, this deployment is not without its challenges, with adversarial attacks being a significant concern. Unlike most existing research that primarily focuses on adversarial attacks in computer vision datasets, their project delves into the practicality of such attacks against ML-based NIDS.
The research paper presents two pivotal contributions. Firstly, it introduces a taxonomy of practicality issues associated with adversarial attacks against ML-based NIDS. Secondly, it investigates the impact of continuous training on adversarial attacks in this context. Notably, the experiments conducted reveal that continuous re-training, even without specific adversarial training, can significantly mitigate the effects of such attacks.
While adversarial attacks pose a potential threat to ML-based NIDS, the research team, under the guidance of Dr. Ashraf Matrawy, emphasizes a critical gap between academic research and real-world practicality in this domain. This underscores the need for increased attention and proactive measures to bridge this gap and fortify the resilience of ML-based NIDS in practical cybersecurity scenarios.
We extend our congratulations to the entire research team for their outstanding achievement and commitment to advancing the field of network security.
For further details and to stay updated on their ongoing research, please visit the website link below.